Multi-Layer Perceptron (MLP) with Keras
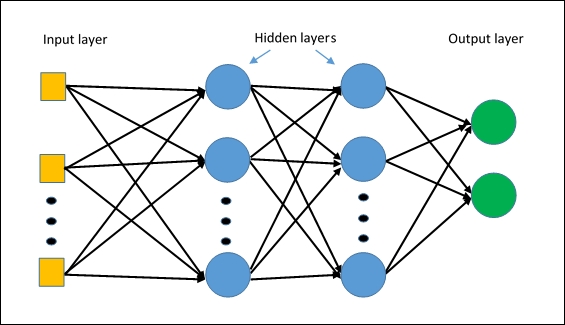
Multi-Layer Perceptron is famous nowadays as we have enough processing power to compute heavy operations. It consists of 3 layers: input, hidden and an output layer. In this post, we will implement the neural network with Keras . Keras is a python deep learning library. In the dataset, we have ASCII representation, where each letter of the alphabet is represented by a matrix of 12x13 binary values. Here, we will train our model and check the analyze the learning curve. So first we will import the required packages and load the data. import numpy as np from keras import models, layers, optimizers, utils from random import randint from keras.layers.core import Activation from keras.layers import Dropout from matplotlib import pyplot as plt dataset = np.loadtxt('pattern1.txt', delimiter=" ") In our data file, we have A to Z letter, so we will transform our data first and then split the train and test data. We’ll also prepare our target column as in